Generative Pretrained Transformer (GPT) | DE

Datasets for GPT models
GPT models can be tailored to the specific needs of the German healthcare system by fine-tuning them with topic-specific data to ensure higher quality results than prompt designs.
In the process, relevant data sets from expert standards, guidelines and social jurisdiction are integrated into the model and the model is re-trained.
In this way, GPT models can provide specific information for healthcare.
ChatGPT by OpenAI is designed to generate text based on patterns it has learned from a large amount of training data.
"The chatbot is based on a computer model that has been trained to process linguistic data using artificial intelligence (AI) methods. It can quickly generate eloquent responses on a wide variety of topics, create entire essays or computer programs, and use language styles such as poems, jokes, or discussions - and in different languages." 1
It uses natural language understanding to analyze queries and its own internal databases to provide answers quickly and efficiently, and accesses third-party data sources such as databases, APIs, and websites to provide more detailed answers.
Training of the models takes place in two phases:
In a first step, the system reads in large quantities of texts largely independently (unsupervised) and forms its parameters from them.
In the second step, human feedback is used to fine-tune the model to its specific task.
This second step requires a detailed knowledge of the German health care system and its regulatory instruments.2
Examples of definitions for durable medical equipment by the Federal Social Court shows deficits of the ChatGPT-3 answers.
Dependence of the results on the quality of the input data
- Data quality and quantity will require access to high-quality, diverse and large datasets.
- Validation of models for input or output monitoring.
- Training with human feedback.
- Extract information from unstructured or semi-structured machine-readable documents.
- Provide data pools and training tools.
System-related limitations
GPT models have so far system- or architecture-related limitations.
Output can only be as good as the input received.
The distorted representation of certain texts, may be reflected in the system's responses and reinforce erroneous content.
Lack of abstractions
- Large amount of training data is processed without being able to form abstractions.
- Bias in the model leading to unfair or unequal recommendations and could exacerbate existing disparities.
- Output that appears plausible, but is not supported by the underlying data.
Health Care
The more parameters ChatGPT includes, the less often fact-oriented answers are given.
ChatGPT has difficulty providing references for its statements.
The distorted representation of certain texts, may be reflected in the system's responses and reinforce erroneous content.
Fine tuning of GPT models
Modifications to GPT models pursue the goal of being used directly in healthcare and increasing the competence of all providers and other stakeholders.
Existing datasets through information extraction improve the ability of GPT models to solve specific tasks in German Health Care.
Lack of transparency and explainability: ChatGPT may not be able to explain its decision-making process, which can make it difficult to identify and correct any bias or errors in the model.
This can reduce trust in the technology and lead to further disparities in health outcomes.
Training will focus on specific data sets, care settings and care scenarios.
Thus, there will be more data available for training machine learning models like ChatGPT, which will enable it to provide more accurate and relevant responses.
Examples of formalized knowledge assets included in training data.
German Federal Social Court on the obligation of rehab providers for rehabilitation procedures.

German Federal Supreme Court on the admissibility of organized discharge management.
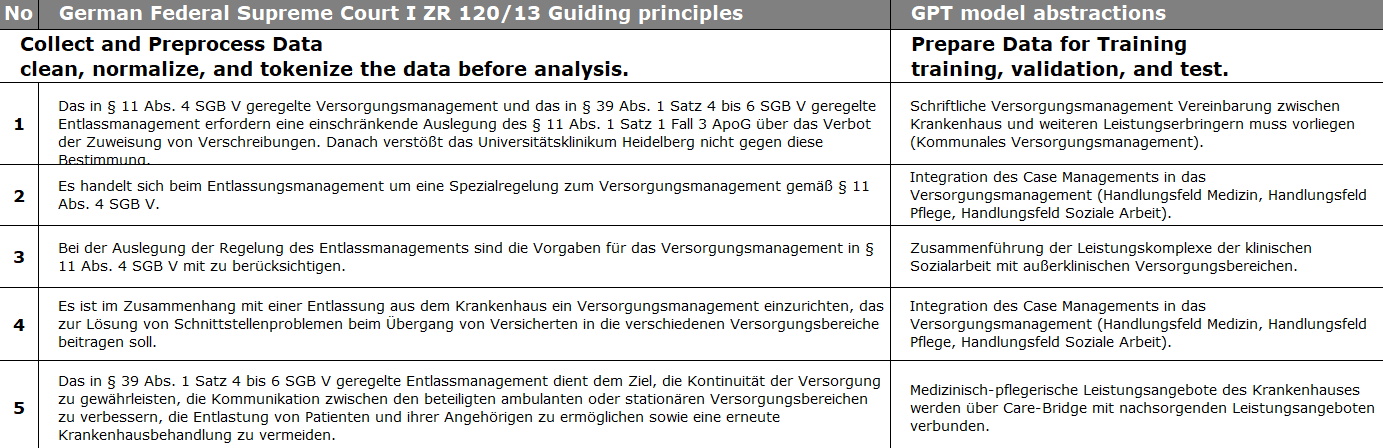
German Network for Quality Development in Nursing (DNQP)3 for the maintenance and promotion of mobility in nursing care.
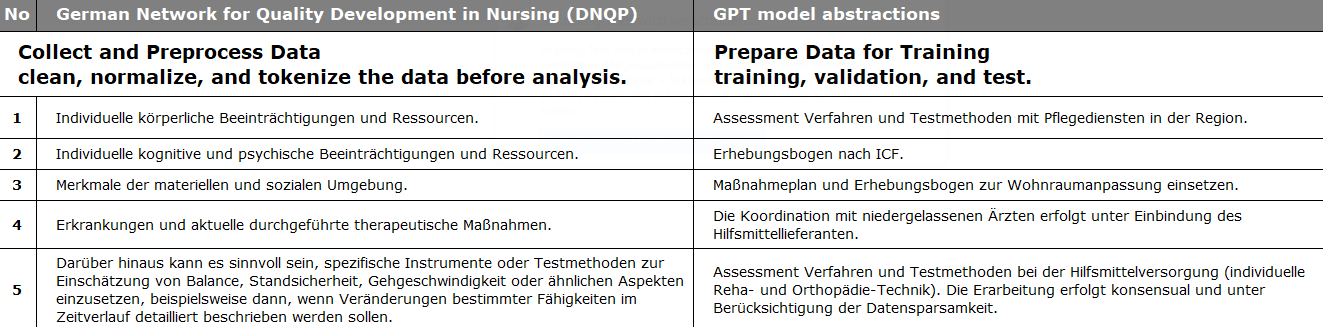
This will lead to more transparency and explainability and will pursue building an effective ecosystem of partners, communities, and platforms.
GPT-models will continue to improve in terms of transparency and explainability, making it easier for healthcare providers and all other stakeholders to understand how the model arrived at its recommendations and to identify and correct any errors.
Conformity assessment procedure based on assessment of the quality management system and of the technical documentation.
In future, healthcare AI systems will have to include provisions on technical documentation and undergo a conformity assessment procedure based on the assessment of a quality management system.4
However, conformity assessment procedures and quality management systems for GPT models need significant further development, especially for the very complex models considered in healthcare.
A training data pool has to be built from unstructured or semi-structured machine-readable documents, maintained according to defined quality criteria, and can be made available to interested organizations.
A no-regrets move is to assemble a cross-functional team, including data science practitioners, health care experts, and business leaders, to think through basic questions.
As a business coach, I could be part of the cross-functional-team and provide consulting and scientific support in the selection of training data pools and validation of GPT models for input and output monitoring.
When designing GPT models for decision support, it must be ensured that results are presented in a way that makes the dangers of automation bias transparent, counteracts them, and emphasizes the need for a reflexive plausibility check of the course of action proposed by the AI system.5
1 Albrecht S.: ChatGPT und andere Computermodelle zur Sprachverarbeitung – Grundlagen, Anwendungspotenziale und mögliche Auswirkungen; Büro für Technikfolgen-Abschätzung beim Deutschen Bundestag, TAB-Hintergrundpapier Nr. 26, Berlin, April 2023.
2 Bundesverband der Unternehmen der Künstlichen Intelligenz in Deutschland e.V.: Large European AI Models (LEAM), Feasibility study - Large AI Models for Germany, Berlin, May 2023.
3 German Network for Quality Development in Nursing (DNQP): Arbeitsunterlagen zur Fachkonferenz zum Expertenstandards nach § 113a SGB XI; Erhaltung und Förderung der Mobilität in der Pflege, Osnabrück: 28. März 2014.
4 European Parliament 2019-2024: Proposal for a regulation of the European Parliament and of the Council on harmonised rules on Artificial Intelligence (Artificial Intelligence Act) and amending certain Union Legislative Acts, COM(2021)0206 – C9 0146/2021 – 2021/0106(COD), Brussels, 9/5/2023.
5 Deutscher Ethikrat: Mensch und Maschine – Herausforderungen durch Künstliche Intelligenz, Stellungnahme, Berlin, 20.03.2023.